It all started with this email that was waiting in my inbox*
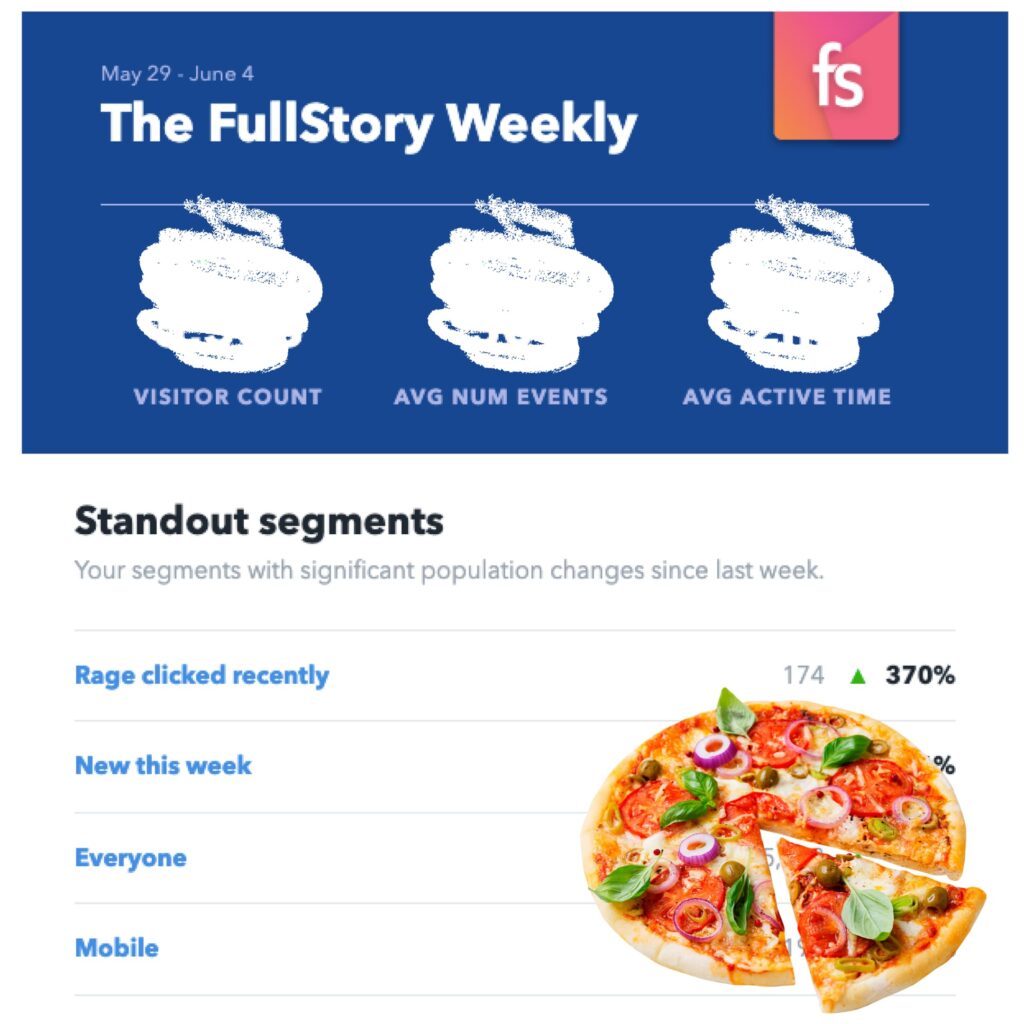
*The pizza is there to hide company data. I was obviously hungry when writing this post.
There is nothing worse for a product manager than starting your work week with a huge disaster (though in some former workplaces, it was just another Monday). I had never seen such a large “rage-click” increase (370%). On top of that, it happened right after our company launched a major new feature. Without further ado, I opened the analytics and checked the data.
Can you guess what happened?
As product managers, we often find ourselves swimming in seas of data and analytics, seeking insights that will guide us towards the correct, data-informed decisions. One of the greatest perks of working in a data-driven company is the plethora of data and analytics tools available. It can feel like a playground. With hours spent browsing and pivoting data graphs, I sometimes feel like a detective solving a puzzling mystery.
However, this alarming email I received was a friendly reminder that data is not infallible. There have been numerous instances where relying solely on data or misinterpreting it has led to poor product decisions. In this post, I’ll share potential pitfalls and examples emphasizing the importance of critical thinking and careful analysis when working with data and analytics tools.
Pitfall 1: Relying on a single metric without validating its accuracy leads to misguided product decisions.
A popular e-commerce company was facing a decline in sales and decided to delve into its data to identify potential causes. They noticed that the “Add to Cart” button on their website had a significantly low click-through rate, leading them to believe it was the culprit. Based on this data, they decided to revamp the entire checkout process to improve conversions.
However, further investigation revealed that the low click-through rate was actually caused by a faulty tracking script, leading to inaccurate data. In reality, customers were adding products to their carts that were not reflected in the analytics. The company’s hasty decision to revamp the checkout process resulted in unnecessary development efforts and failed to address the underlying issue.
Returning to the “rage click” email I received… My inquiry indicated that the source of this newly profound “rage” was, in fact, several data events that were automatically added by one of our analytics tools following a new feature release. Therefore, enthusiastic early adopters were mistakenly reported in the analytics app as “rage clicks.”
Pitfall 2: Early user testing is not enough. The collected data set should be comprehensive enough and tested on real, diverse users, in their natural environment and context of use.
Microsoft’s Clippy, the animated paperclip assistant introduced in Office 97, was intended to help users navigate the software more easily. However, Clippy’s intrusive and often irrelevant suggestions annoyed users rather than assisted them. Microsoft’s decision to include Clippy was based on early user testing that indicated positive responses. However, when the product was released to a larger user base, it became evident that the initial test results did not accurately represent the majority of their users’ preferences and needs.
Google Wave, launched in 2009, was positioned as a revolutionary collaboration tool that combined messaging, document sharing, and real-time editing. Google believed it would transform communication and collaboration. However, despite the hype and initial positive feedback from early testers, the product failed to gain traction and was eventually discontinued. It turned out that the early feedback and data were skewed due to the enthusiasm of tech-savvy testers, which did not reflect the broader user base’s needs and preferences.
Pitfall 3: It’s crucial to scrutinize the underlying causes behind observed metrics to ensure they align with the desired outcomes and are not influenced by external factors.
In 2012, Target faced significant backlash when it sent pregnancy-related coupons to a teenage girl, inadvertently revealing her pregnancy to her family. Target analyzed purchasing patterns and customer data to identify potential pregnancy predictors, such as certain products commonly bought by pregnant women. However, the algorithm misinterpreted the data in this case, leading to an inappropriate and unintended outcome. This event highlighted the importance of considering privacy and ethical implications when working with sensitive customer data.
Several years ago, I opened an online store selling LGBT-themed t-shirt designs. Young, excited, and filled with dreams, I started a store-launch marketing campaign. I tracked user interactions and time spent on the website as key metrics to measure my marketing campaign success. After a few days of spending my life savings on Facebook ads, I observed a substantial increase in user interactions. Right before I opened my bottle of champagne, upon closer inspection, I realized that the increased interactions were primarily due to a rise in spam bot activities and hacking attacks of anti-LGBT activists who targeted my store, rather than genuine user engagement.
Pitfall 4: Data-driven decisions should be complemented by qualitative research and customer feedback to gain a holistic understanding of user behavior.
An online retailer analyzed their conversion rate for different user demographics and discovered that one particular age group had the lowest conversion rate. Based on this finding, they decided to tailor their marketing campaigns exclusively toward other age groups, assuming it would yield better results.
However, further investigation revealed that the low conversion rate was not due to disinterest from the specific age group but rather because of a poorly optimized checkout process that presented technical difficulties for that demographic. The data, though accurate, failed to provide the complete picture, leading the company to overlook an untapped market segment.
Netflix discovered the hard way that relying solely on user ratings for predicting watch behavior was not sufficient. In 2014, Netflix famously announced a $1 million prize for anyone who could improve their recommendation algorithm by 10%. However, the winning team discovered that while the algorithm was successful in recommending films users were likely to rate highly, it failed to predict whether users would actually watch those films. This oversight led to a flawed understanding of user behavior and resulted in inaccurate recommendations. Netflix learned that relying solely on user ratings for predicting watch behavior was not sufficient. Qualitative research would have prevented this outcome.
In conclusion:
We are all human, and so we are all bound to make mistakes.
With A/B testing, diversifying our data resources and tested populations, and challenging our assumptions, we can reduce the risk of making costly product mistakes when following data blindly. In order to mitigate the risks posed by these traps, it is useful to occasionally reflect and remind ourselves of these 4 important pitfalls.
What data-related mistakes have you encountered? Have you heard of other interesting examples? I’d love to hear!